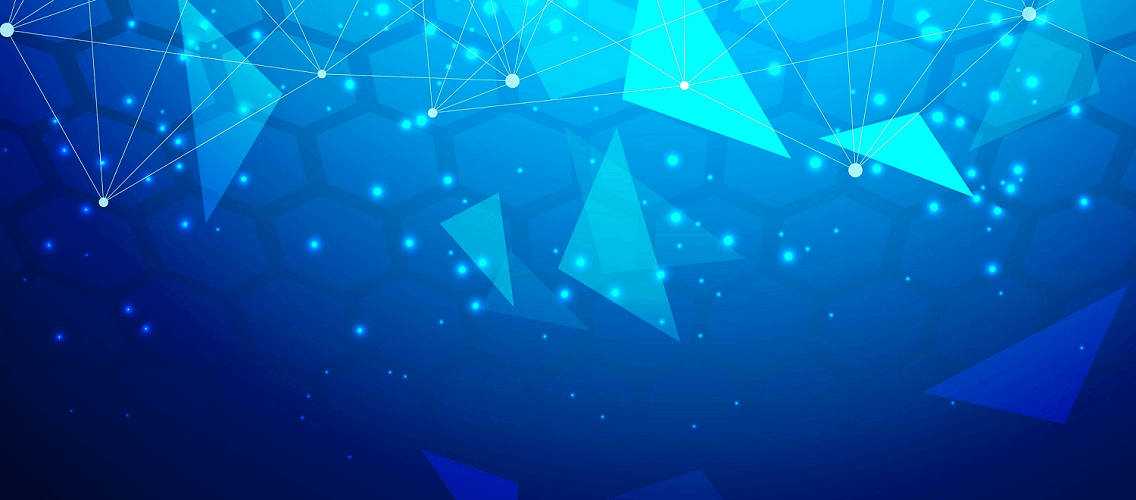
Camunda 7 Enterprise End of Life (EoL) Extension Announcement
In today’s fast-paced world of data analytics and AI, optimizing your data infrastructure is key to unlocking valuable insights and driving innovation.
Sometimes the problem with artificial intelligence (AI) and automation is that they are too labor-intensive. That sounds like a joke, but we’re quite serious. Traditional AI tools, especially deep learning-based ones, require huge amounts of effort to use. You need to collect, curate, and annotate data for any specific task you want to perform. This is often a very cumbersome exercise that takes significant amount of time to field an AI solution that yields business value. And then you need highly specialized, expensive and difficult to find skills to work the magic of training an AI model. If you want to start a different task or solve a new problem, you often must start the whole process over again—it’s a recurring cost.
But that’s all changing thanks to pre-trained, open source foundation models. With a foundation model, often using a kind of neural network called a “transformer” and leveraging a technique called self-supervised learning, you can create pre-trained models for a vast amount of unlabeled data. The model can learn the domain-specific structure it’s working on before you even start thinking about the problem that you’re trying to solve. This is usually text, but it can also be code, IT events, time series, geospatial data, or even molecules.
Starting from this foundation model, you can start solving automation problems easily with AI and using very little data—in some cases, called few-shot learning, just a few examples. In other cases, it’s sufficient to just describe the task you’re trying to solve.
Some foundation models for natural language processing (NLP), for instance, are pre-trained on massive amounts of data from the internet. Sometimes, you don’t know what data a model was trained on because the creators of those models won’t tell you. And those massive large-scale datasets contain some of the darker corners of the internet. It becomes difficult to ensure that the model algorithms outputs aren’t biased, or even toxic. This is an open, hard problem for the entire field of AI applications. At IBM, we want to infuse trust into everything we do, and we’re building our own foundation models with transparency at their core for clients to use.
As a first step, we’re carefully curating an enterprise-ready data set using our data lake tooling to serve as a foundation for our, well, foundation models. We’re carefully removing problematic datasets, and we’re applying AI-based hate and profanity filters to remove objectionable content. That’s an example of negative curation—removing things.
We also do positive curation—adding things we know our clients care about. We’ve curated a rich set of data from enterprise-relevant domains—finance, legal and regulatory, cybersecurity, sustainability data. Datasets like this are measured in how many “tokens”—think of those as words or word parts—that we’re including. We’re targeting a 2 trillion token dataset, which would make it among the largest that anyone has assembled.
Next, we’re training the models, bringing together best-in-class innovations from the open community and those developed by IBM Research. Over the next few months, we’ll be making these models available for clients, alongside the open-source model catalog mentioned earlier.
Harnessing the power of foundation models at scale
Foundation models represent a paradigm shift in AI, one that requires not only a new technical stack to allow hybrid cloud environments to flourish, but also fundamentally new user interactions that harness the power of these models for enterprise. Coming soon, our enterprise-ready next-generation AI studio for AI builders, watsonx.ai has two tools for generative AI capabilities powered by foundation models to help bridge this gap for clients: a Prompt Lab and a Prompt Tuning Studio.
The Prompt Lab
The Prompt Lab enables users to rapidly explore and build solutions with large language and code models by experimenting with prompts. Prompts are simple text inputs that effectively nudge the model to do your bidding with direct instructions. Prompts can also include a few examples to guide the model towards the exact behavior you’re looking for.
With language models, all you have to do is write the instructions in natural language. It usually takes a certain amount of trial and error to craft the right prompt that can enables the model to generate the desired result, a new field called prompt engineering. For instance, within the Prompt Lab, users can leverage different prompts for both zero-shot prompting and few-shot prompting to accomplish different tasks such as:
With Prompt Lab, practically anyone can harness the power of foundation models for enterprise use cases. Engineers and developers can also use our APIs to embed these capabilities into external and internal applications. We’re actively working on more enhanced developer experience that offers useful libraries and code samples.
The Tuning Studio
With the watsonx.ai Tuning Studio, users can further customize foundation model behavior using a state-of the art method that requires as few a 100 to 1,000 examples. By using advanced prompt tuning within watsonx.ai, you can efficiently create and deploy a foundation model that is customized to your data.
Tuning can be useful to adapt existing models to domain-specific tasks (i.e., learn new tasks). It also allows enterprises to harness their proprietary data to differentiate their applications.
In the Tuning Studio, all you have to do is specify your task and provide labelled examples in the required format. Once the model training is complete, you can deploy the model and use it in both the Prompt Lab and via an API.
What are we doing ahead of the release?
As we gear up towards our broader watsonx.ai release in July, we’re actively seeing new use cases being built out through our Tech Preview program. We are investing in a roadmap of state-of-the-art tooling to efficiently customize models with proprietary data. We’re improving our Prompt Lab with interfaces that help novice users construct better prompts and guide the models to providing the right answers more quickly.
In addition, we recently open-sourced a preview of our python SDK and announced a partnership with Hugging Face to integrate their open-source libraries into watsonx.ai. The foundation model capabilities within watsonx.ai fit into a greater data and AI platform, watsonx, alongside two other key pillars watsonx.data and watsonx.governance. Together, watsonx offers organizations the ability to:
Message replication configuration trade-offs
IBM Global Mailbox - Technical overview
Browse Categories
Share Blog Post
In today’s fast-paced world of data analytics and AI, optimizing your data infrastructure is key to unlocking valuable insights and driving innovation.
In today’s fast-paced world of data analytics and AI, optimizing your data infrastructure is key to unlocking valuable insights and driving innovation.
In today’s fast-paced world of data analytics and AI, optimizing your data infrastructure is key to unlocking valuable insights and driving innovation.
At Pragma Edge, we are a forward-thinking technology services provider dedicated to driving innovation and transformation across industries.
Cookie | Duration | Description |
---|---|---|
cookielawinfo-checkbox-analytics | 11 months | This cookie is set by GDPR Cookie Consent plugin. The cookie is used to store the user consent for the cookies in the category "Analytics". |
cookielawinfo-checkbox-functional | 11 months | The cookie is set by GDPR cookie consent to record the user consent for the cookies in the category "Functional". |
cookielawinfo-checkbox-necessary | 11 months | This cookie is set by GDPR Cookie Consent plugin. The cookies is used to store the user consent for the cookies in the category "Necessary". |
cookielawinfo-checkbox-others | 11 months | This cookie is set by GDPR Cookie Consent plugin. The cookie is used to store the user consent for the cookies in the category "Other. |
cookielawinfo-checkbox-performance | 11 months | This cookie is set by GDPR Cookie Consent plugin. The cookie is used to store the user consent for the cookies in the category "Performance". |
viewed_cookie_policy | 11 months | The cookie is set by the GDPR Cookie Consent plugin and is used to store whether or not user has consented to the use of cookies. It does not store any personal data. |
Thank you for submitting your details.
For more information, Download the PDF.
Thank you for registering for the conference ! Our team will confirm your registration shortly.
Invite and share the event with your colleaguesÂ
IBM Partner Engagement Manager Standard is the right solution
addressing the following business challenges
IBM Partner Engagement Manager Standard is the right solution
addressing the following business challenges
IBM Partner Engagement Manager Standard is the right solution
addressing the following business challenges